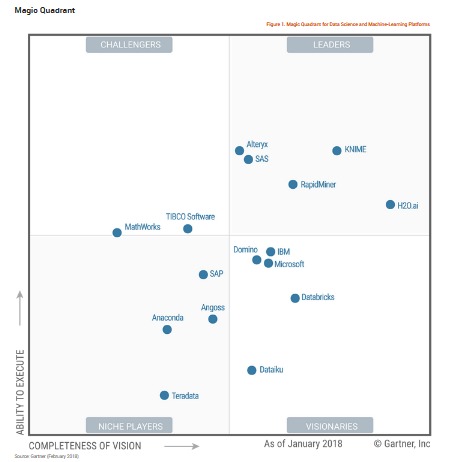
-
05 Sep
-
Magic Quadrant for Data Science and Machine-Learning Platforms - Part 2
Readers should know that:
- Gartner invited a diverse mix of data science platform vendors to participate in the evaluation process for potential inclusion in this Magic Quadrant, as data scientists have different preferences for UIs and tools. Some prefer to code data science models in Python or R; others favor Scala or Apache Spark; some like to run data models in spreadsheets; others are more comfortable building models by creating visual pipelines via a point-and-click UI. Tool diversity is an important characteristic of this market.
- The wide range of products available offers a breadth and depth of capability and varied approaches to developing and deploying models. It is therefore important to evaluate your specific needs when assessing vendors. A vendor in the Leaders quadrant, for example, may not be your best choice. For an extensive review of the functional capabilities of each platform, see "Critical Capabilities for Data Science and Machine-Learning Platforms."
- Open-source platforms are excluded from this Magic Quadrant if they have no vendor supporting them as commercially licensable products. Commercially licensed open-source platforms are included. We also recognize the growing trend for commercial platforms to use open-source libraries and content. Open-source solutions represent an opportunity to get started with data science and machine learning with little upfront investment (see Note 1).
- Artificial intelligence (AI) is the subject of considerable hype, but cannot be ignored. Data science is undoubtedly a core discipline for the development of AI. Machine learning is a core enabler of AI, but not the whole story. Machine learning is about creating and training models; AI is about using models to infer conclusions under certain conditions. A self-driving car, for example, has machine-learning capability, but its AI requires much more than that.
The diversity of data science platforms largely reflects the diverse types of data scientist who use them. This Magic Quadrant is therefore aimed at a variety of audiences:
- Line of business (LOB) data science teams. Typically, these are sponsored by their LOB's executive and charged with addressing LOB-led initiatives in areas such as marketing, risk management and CRM. They focus on their own priorities. Levels of collaboration with other LOB data science teams vary.
- Corporate data science teams. These have strong and broad executive sponsorship, and can take a cross-functional perspective from a position of enterprisewide visibility. In addition to supporting model building, they are often charged with defining and supporting an end-to-end process for building and deploying data science and machine-learning models. They often work in partnership with LOB data science teams in multitier organizations.
- Adjacent, "maverick" data scientists. These are typically one-off scientists in various LOB units. They tend to work independently on "point" solutions and usually strongly favor open-source tools, such as Python, R and Apache Spark. They rarely collaborate much with other data scientists in their organization.
- Holders of additional roles, such as citizen data scientist, data engineer and application developer. They need to understand the nature of the data science and machine-learning market, and how it differs from, but complements, the analytics and business intelligence (BI) market (see "Magic Quadrant for Analytics and Business Intelligence Platforms").
Magic Quadrant
Figure 1. Magic Quadrant for Data Science and Machine-Learning Platforms
Sourced by: https://www.gartner.com/doc/reprints?id=1-4RNH03T&ct=180222&st=sb&utm_content=buffer21830&utm_medium=social&utm_source=twitter.com&utm_campaign=buffer
- 05 Sep, 2018
- 168Solution Public Class